Demystifying Artificial Intelligence (AI) in Healthcare
Stacey J. Drubner, JD, LICSW, MPH
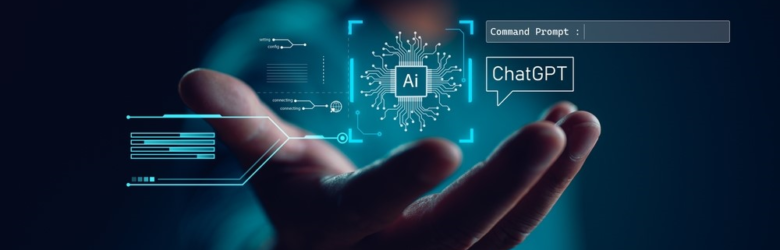
EAP Ask the Experts from The AI in Medicine Program (AIM) at Mass General Brigham (MGB): Hugo Aerts PhD, Director (AIM) & Danielle Bitterman, M.D. Faculty Member (AIM) & Radiation Oncologist (Brigham and Women’s Hospital/Dana-Farber Cancer Institute)
Discussions about artificial intelligence (AI) are everywhere – in the media, on Capitol Hill, and at MGB. Some in our system were early adopters, some are current innovators, and others may have no idea what AI is or why it matters. At some point, all stakeholders in healthcare will interface with AI. While not everyone needs to be an expert, at a minimum, we should all develop a baseline knowledge of AI, and an understanding of potential benefits and the need to monitor for efficiency, quality, and safety.
The amount of information and the pace of development can make AI seem confusing and overwhelming. For this feature, we turned to system experts, and Dr. Hugo Aerts and Dr. Danielle Bitterman, from the AIM Program, to help deconstruct AI in healthcare. Full disclosure: this article was drafted the “old-school way,” without AI.
What is AI?
AI is a broad field with many components. Danielle offers a simple general description: AI is Anything that teaches a computer to carry out human-like tasks. For example, identifying patients at risk for developing complications from their vital signs. Hugo adds that AI excels in recognizing complex patterns in data and information that can make medicine more effective and efficient.
Examples include:
- Natural language processing – teaching a computer to understand human language and text, so it can understand and process the world. This includes programs such as ChatGPT
- Machine and deep learning – Computers are programmed to train themselves about the data they are looking at and to analyze generalities. Like humans, the computer learns from the environment. Examples of this are AI programs that detect cancers in medical imaging data or predict treatment responses from EHR data
- Robotics – Robots are built and programmed to perform specific duties
Potentially Relevant Impact Areas for AI in Healthcare
There are more possibilities than we can cover in this overview, but we review some key areas below.
Administrative processes and efficiencies
The earliest, most noticeable and advanced areas of impact are in the administrative space. Some examples include:
- Coding
- Billing
- Documentation
- Improved and targeted scheduling to match patients with the right provider
- Monitoring & forecasting of hospital capacity
Data Management
Recent advances in model architecture and computational ability allow for more efficient management of large amounts of data. This can support clinical care, quality improvement, patient experience and research.
- Mining and extraction of health record data to get targeted information from notes
- Use of clinic data to quantify current disease /response, identify treatments and predict future diseases
- More robust data in areas where there are current gaps, such as with side effects of cancer treatments, which are not always represented or reliably reported in current registries
- Ability to more easily identify patients with social determinants of health (SDOH) issues to increase supports
Diagnostics and Care Support
Many diagnostic and outcomes AI initiatives are currently in use, and even more are in the research phase. This part of AI will certainly evolve much more in the future.
Since Hugo and Danielle work in the oncology space, they are most familiar with projects related to this specialty area. For example, AI is used to detect abnormalities in radiology studies or to provide reliable automated planning for radiation (currently being used at MGB). This allows clinicians to identify the most accurate location, size, contour and dose for radiation treatment and to review and make adjustments throughout treatment. This helps to ensure the most targeted, cost-effective care, and may prevent unnecessary radiation impacts. Since more than 50% of cancer patients receive radiation as part of their treatment plan, this advance is quite meaningful.
There are too many clinical and research projects to list but here are examples from across our system:
- AI & Detecting Alzheimer’s Disease
- AI & Lung Cancer
- Using AI to Estimate Lung Disease Mortality from X-rays
- Auto-contouring for Prostate Cancer
- Predicting Cardiovascular Risk from Standard-of-care Imaging using AI
- Using a Deep Learning-based Model to Identify Heart Defects
- New AI Technology Integrates Multiple Data Types to Predict Cancer Outcomes
Provider & Patient Supports
An important component to the success of AI is the buy-in from patients and providers. They are unlikely to support or accept innovations if they don’t see the value in these changes. A recent Pew Research Survey reported that 60% of U.S. adults say they would feel uncomfortable if their own healthcare provider relied on artificial intelligence to do things like diagnose diseases and recommend treatments.
Hugo predicts that using new AI technologies will be a “win-win” for both patients and providers because administrative efficiencies will allow clinicians to spend more time on what matters – communicating with and providing care to patients. This can improve the patient-provider relationship.
Danielle adds that including patients and providers (not just the tech-savvy) in the conversation is an important step toward successful implementation. Below, we discuss some of the ways that AI can make healthcare better for providers and patients going forward.
For Providers
Medicine has become more complex with a growing knowledge base, medical advances, and related side effects. The care population is living longer and has more co-morbidities. Data sources are overwhelming, and it’s challenging to incorporate all available information. As mentioned above, AI has the potential to minimize provider burden via managing multiple data variables and streamlining administrative tasks. This includes but is not limited to:
- Simplifying the complex “dataverse”
- Providing patient and population-specific information
- Synthesizing and communicating data between specialists and generalists
- Managing the documentation burden
- Increasing access to information to keep people out of ED/inpatient
- Offering the potential for scalability
-Hugo indicates that AI developed in one clinic can often easily be deployed elsewhere in the MGB system or in other clinics, with fewer resources
For Patients
- The potential for improved and faster access to information and responses to questions
- Improved converting/translating of health record data
- Streamlined translation across languages
- Easier to find the right provider match for specific clinical needs
- Better and more direct communication options
- Benefits from providers who are better able to focus on patients when administrative burdens are mitigated
Barriers and Caveats
While Danielle and Hugo are extremely passionate about AI, they are equally motivated to ensure that AI in research and clinical settings is rigorously evaluated. Any technical revolution requires trial and error processes. We shouldn’t sacrifice effectiveness and privacy for convenience. Below are some of the recommendations they identified:
- Hold AI technology to the same quality standards that we do for other medical interventions
- Search for evidence that it improves outcomes and professional and patient experience
- Include benchmarking and be open to learning about what we don’t know
- Be very careful with AI tools
-For example, there are studies in our system that show positive results with ChatGPT, but Danielle and her colleagues have a recent publication that uncovered some deficits with this program. AI answers to common questions associated with cancer diagnoses had about a 1/3 deviation from industry standards - Continually monitor to make sure AI tools are doing what they are supposed to be doing
- Place AI where it makes sense to do so
- Be mindful of “automation bias” where people just accept what the computer develops
- Ensure that there is population transferability and accommodation
- Develop an infrastructure to monitor the models. Avoid silos and unnecessary duplication
- Centralize models to minimize compromising PHI
- Take measures to make sure data is secure and accessible only within MGB
- Be transparent and respect patient and provider autonomy via careful consent
- Articulate when and how AI models and data will be used
- Remember that AI is meant to augment, not replace traditional care; we don’t want to lose the value of the therapeutic nature of in-person clinical contact and human reasoning
How to Learn More about and Get Involved in AI in Healthcare
The discussion in this feature is meant to provide an introduction to AI. There are many ways you can continue to learn about or work with AI. Hugo suggests looking to your department for support or connecting with your specialty area professional organizations. Otherwise here are some resources related to AI in healthcare:
AIM – MGB AI Academic Research Program
Artificial Intelligence at Mass General Brigham (sharepoint.com)
MGB – Artificial Intelligence for Clinicians Lecture Series
The Alliance for Artificial Intelligence in Healthcare
Journal – Artificial Intelligence in Medicine
Help from the EAP
The EAP offers free and confidential services for employees and immediate family, household members. EAP records are separate from medical and HR records. Contact the EAP at 866-724-4327 or request an appointment via our online form for confidential assistance. In-person appointments are available at the following locations. Phone or Video (Zoom) appointments are available from all locations.